Deep learning is a subset of machine learning that involves Artificial Neural Networks to solve complex problems by mimicking human behavior. Neural networks are designed in such a way that they resemble the human brain’s capabilities and can learn complex patterns and make predictions by analyzing large amounts of data.
In deep learning, the model is trained on massive amounts of data, and this data teaches the model how to recognize patterns and make predictions.
In this article, we will discuss the common applications of deep learning in AI.
Table of Contents
Which are common applications of Deep Learning in Artificial Intelligence?
Following are some of the common practical applications of Deep Learning in Artificial Intelligence:
Natural Language Processing (NLP)
Natural Language Processing assists computers or machines to interpret, manipulate, and comprehend human language. In simple words, NLP carries out the interaction between human language and computers.
Deep Learning algorithms can automatically extract meaning from the text and bring a radicle growth in NLP. Deep Learning is used to understand, process, and generate human language. It is used in different applications, such as sentiment analysis, text summarization, language translation, question answering, chatbots, etc.
Healthcare
Deep Learning has revolutionized healthcare by developing certain applications that can improve diagnostics, treatment, and patient outcomes. These applications assist in diagnosing diseases from medical images like CT scans, X-rays, and MRIs, predicting patient outcomes, and drug discovery.
These algorithms analyze the medical data to identify patterns and trends that help in the early detection of certain critical diseases like cancer, blood clots, diabetes, etc., that might not be visible to the naked eye and also assist in the treatment recommendation.
Fraud Detection
Deep Learning algorithms help in recognizing and preventing fraudulent transactions, such as credit card fraud, net banking fraud, etc., and are used in a variety of applications, such as banking and insurance.
These models are trained on the large dataset of banking transactions with fraud cases and learn the patterns of fraud and thus help in future fraud prevention. It can make fraud more predictable and, hence, avoidable.
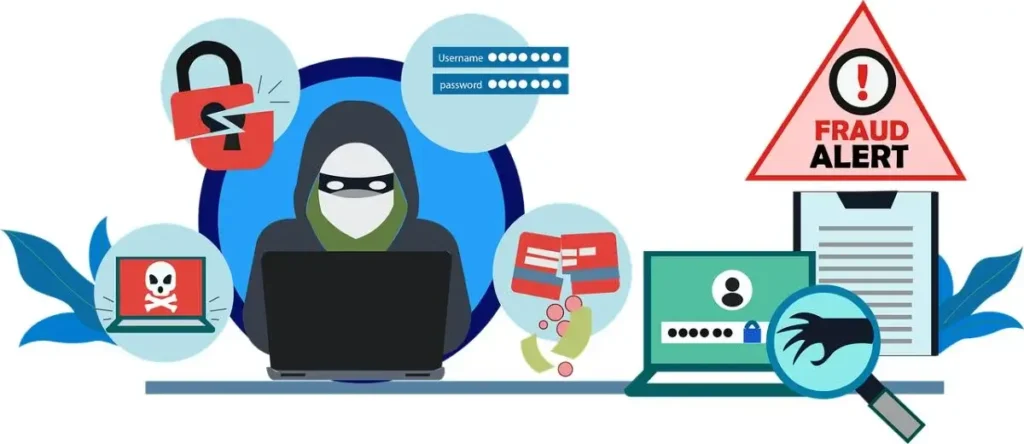
Finance
These algorithms are used in a variety of financial services applications such as stock market prediction, risk management, credit risk assessment, fraud detection, etc.
Deep Learning applications are used by many financial organizations in decision-making and risk assessment that help the organizations prevent financial losses and increase financial growth.
These models are trained on large financial datasets to learn the patterns and correlations and help financial organizations predict future risks, business opportunities, and profits.
Recommendation Systems
These algorithms are trained on recommendation datasets, which help in recommending products, services, and content to users. Online platforms use deep learning applications to analyze user preferences and behavior and provide personalized recommendations for movies, music, products, etc. on e-commerce and OTT platforms.
Autonomous Vehicles/ Self Driving Cars
Deep learning models are trained on large datasets of images and driving data, which enables the model to learn driving patterns and object identification, like identifying pedestrians, road signs, and other vehicles.
It helps self-driving cars by enabling them to recognize and respond to their environment, thus enabling them to make wise decisions, object identification, and guarantee the safety of passengers and pedestrians.
Virtual Assistants
Virtual assistants are applications capable of performing human tasks such as scheduling appointments, sending emails, etc. Virtual assistants understand natural language voice commands from humans and complete their tasks.
These models are trained on the large datasets containing the user experiences and they learn the command patterns that help the virtual assistants provide a better user experience based on past experiences. It assists the virtual assistant in understanding the user command effectively and responding accordingly.
Gaming and Virtual Reality
Deep learning has revolutionized the gaming and virtual reality industries by improving graphics rendering, game AI, character animation, and enhancing player experiences.
These algorithms help adjust and customize the gameplay experience by creating more intelligent game characters and enhancing character behaviors and real-time motions.
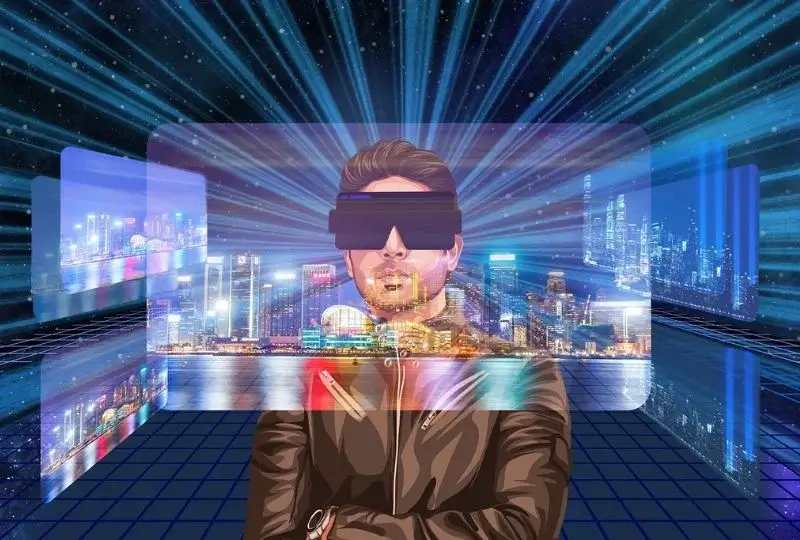
Image Recognition and Computer Vision
These models are trained on large image and video datasets, which helps the model identify and classify objects in images and videos. This improves the application efficiency of image processing tasks such as object recognition, facial recognition, and scenario understanding.
It is used in a variety of applications, such as self-driving cars, medical image analysis, facial recognition, etc.
Robotics
Deep learning is used to control and navigate robots to perform human-like tasks. These algorithms enhance robots to interact with surroundings, identify and grasp objects, interact with humans, and learn and improve their skills.
For example, Deep learning assists the robots to sense the obstacles in their path and pre-plan their journey.
It is used in a variety of applications, such as manufacturing, healthcare, IT, etc.
Chatbots
A chatbot is a computer program that uses artificial intelligence to chat online via text and solve customer issues.
Deep learning algorithms are used in chatbots so that they can interact with humans and understand and respond to their queries in natural language that feels natural and human-like. It helps the chatbots recognize the user’s intent and generate responses accordingly.
Chatbots are widely used as customer care representatives these days by many industries, such as e-commerce, education, healthcare, etc.
Entertainment
Deep learning algorithms are used to create realistic 3D characters and animations and improve the special effects quality.
Also, it helps entertainment companies like Disney, Amazon, YouTube, Netflix, etc. to give relevant songs, web series, movies, etc., to enhance the customer experience.
These models are trained on large datasets based on a person’s interests, which assists streaming companies in suggesting movies, web series, and video recommendations to their users.
Music Composition
Deep learning algorithms are used in applications that can compose music automatically. These models are trained on large music datasets, which helps them learn the notes, structures, and patterns of music and compose music independently.
These applications use LSTM (Long Short Term-Memory) neural networks to compose music by generating musical sequences.
Drug Discovery
Deep learning models are used to accelerate the drug discovery process. These algorithms can be designed in such a way that they can predict molecular interactions and chemical compounds, which helps in discovering new drugs that are beneficial for healthcare.
Cybersecurity
Deep learning is also used in cybersecurity for the detection and prevention of threats, malware, and intrusions, which helps organizations detect and respond to different types of threats effectively and quickly and thus prevent damage.
These algorithms help in developing malware detection systems that can identify patterns that may indicate the presence of malware, which helps in preventing and detecting malware attacks.
Climate Modelling
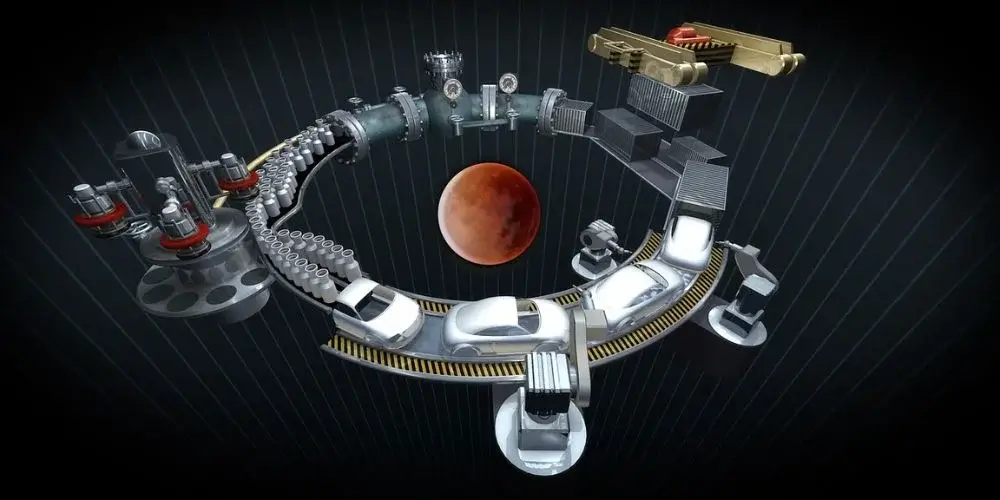
These algorithms are used to build applications that help in weather forecasting, climate data analysis, and understanding climate systems. These applications are trained on large datasets that can predict future weather conditions such as heavy rainfall, intensive wave heat, thunderstorms, etc.
Agriculture
Deep learning can help farmers maximize production and reduce costs with the help of crop monitoring and analyzing parameters such as temperature, soil moisture, weather conditions, soil nutrient level, etc.
These algorithms can be used in applications for crop monitoring and precision farming that help farmers make better decisions about crop cultivation and achieve better efficiency.
Speech Recognition
Deep learning algorithms are used to build accurate speech recognition systems that are used to recognize and transcribe human speech. These models are trained on large speech datasets that enable them to learn the structure and patterns of speech. These models are used in creating a variety of applications, such as voice assistants, dictation software, and transcription services.
Image Captioning
In image captioning, a textual description of an image is generated. These algorithms help improve image captioning systems by automatically generating textual descriptions of images with great accuracy.
Social Media Analysis
Deep learning models are trained on large social media datasets, which helps in identifying and analyzing trending topics for users, understanding user behavior, and performing sentiment analysis on social media platforms.
These models suggest advertising according to user interest on social media platforms.
Advertising
Deep learning helps organizations suggest targeted advertisements to users based on the user’s interests and behavior. It helps advertisers and publishers increase their advertising campaigns.
It increases the user’s experience by ensuring the advertisements they see are more relevant to them than non-relevant advertisements.
Language Translations
Deep learning algorithms are used to build translation applications that are highly accurate and efficient. These models are trained on large text datasets, which enables them to learn translation rules and increase the translation quality.
Conclusion
Deep learning is already being used in many industries, including manufacturing, cybersecurity, healthcare, entertainment, e-commerce, agriculture, and many others. It has revolutionized organizations by making tasks easier.
Above are some of the applications of deep learning in Artificial Intelligence that we have discussed, and it will continue to expand across various industries and organizations in the coming future.
If you wish to learn about deep learning in detail, you can visit our detailed article on it – What is Deep Learning?
Frequently Asked Questions
Q1: What is deep learning?
A: Deep learning is a subset of machine learning that involves Artificial Neural Networks to solve complex problems by mimicking human behavior. Neural networks are designed in such a way that they resemble the human brain’s capabilities and can learn complex patterns and make predictions by analyzing large amounts of data.
In this, the model is trained on massive amounts of data, and this data teaches the model how to recognize patterns and make predictions.
Q2: How Does Deep Learning Works?
A: It works by using artificial neural networks. Neural networks are designed in such a way that they resemble the human brain’s capabilities and mimic human behavior. Each neuron receives input from other neurons and sends output to other neurons.
Q3: What are common applications of Deep Learning in Artificial Intelligence?
A: There are many applications of deep learning in AI like NLP, Fraud Detection, Autonomous Vehicles, Chatbots, Drug Discovery ec.